Are you ready to discover the full power of ChatGPT? Imagine having a language tool that can help you in many ways – from answering questions and creating fun content to giving personalised advice. This is where prompt engineering comes in – a powerful method that makes Chat GPT work better by carefully creating prompts and instructions.
In this blog post, we explore the exciting world of prompt engineering, looking at its basics, techniques, best practices, and advanced strategies, even to create a chatbot. If you're interested in what language models can do, this article is your guide to getting the most out of ChatGPT-4 Turbo.
Get set to learn the secrets of prompt engineering and change how you use ChatGPT. Let's dive in!
Table of Contents
A brief overview of ChatGPT
Prompt Engineering fundamentals
What are the techniques for effective prompt engineering?
What are prompt engineering best practices?
What are advanced prompt engineering strategies?
Case studies
ChatGPT prompt engineering for developers
Final thoughts
A brief overview of ChatGPT
First, let's take a moment to understand what ChatGPT is all about. Developed by OpenAI, Chat GPT is an advanced language model trained on vast text data, enabling it to generate human-like responses to various prompts. Its ability to understand and develop coherent text has captured the attention of professionals across industries.
And now there's Chat GPT-4 Turbo which appears to be an updated version of the GPT-4 model by OpenAI. Here are some key points about it:
- More Capable: GPT-4 Turbo is described as more capable, suggesting improvements in its ability to understand and generate text.
- Updated Knowledge: It has knowledge of world events up to April 2023, which means it has been trained on data that includes more recent information than previous models.
- Larger Context Window: The model boasts a 128k context window, allowing it to process and remember much larger chunks of text in a single instance. This is significant for complex tasks that require an understanding of long documents or conversations.
- Cost-Effective for Developers: It is mentioned to be three times cheaper for developers, which could make it more accessible for a broader range of applications and services.
- Integration with Tools: There might be new tools or APIs specifically designed to work with GPT-4 Turbo, enhancing its usability for creating chatbots and other interactive applications.
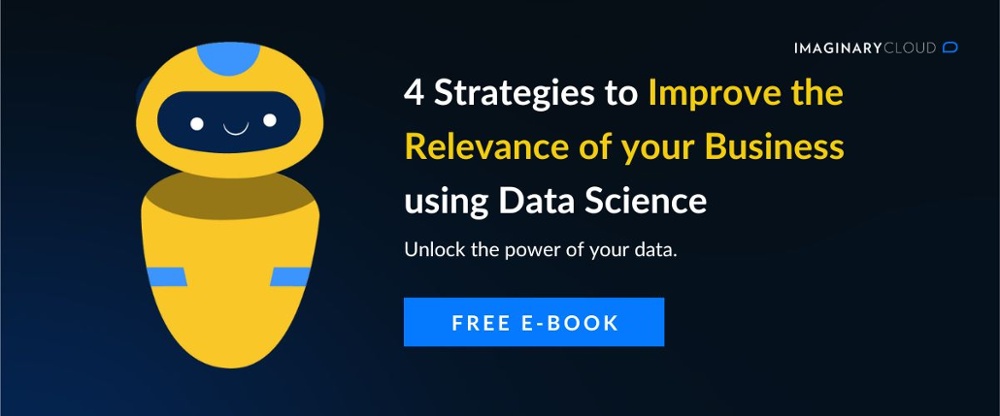
Prompt Engineering fundamentals
Prompt engineering is critical to maximising the usefulness of OpenAI's powerful language model, ChatGPT. In this section, we'll look at the basics of it, including its definition, the role of prompts in engaging with ChatGPT, and the many elements that drive prompt selection.
What is Prompt Engineering?
The strategic process of planning and generating prompts to elicit desired responses through ChatGPT is called prompt engineering. It entails meticulously creating instructions and inputs that control the model's behaviour and shape the quality and relevance of the model's generated output.
Its importance resides in its capacity to improve ChatGPT's capabilities and adjust its responses to specific activities or objectives. Users can successfully explain their intent and elicit accurate and contextually appropriate information from the model by offering well-crafted suggestions.
Why are prompts essential to ChatGPT interaction?
Prompts are essential in the interaction between users and ChatGPT. They give the required context for the model to create relevant responses and act as the starting point for talks. Users can direct ChatGPT towards desired outcomes by structuring instructions with clarity and accuracy.
According to research, prompt engineering considerably impacts the performance of language models. Well-designed prompts can help prevent harmful or biased outputs, boost the accuracy of generated responses, and allow more control over the model's behaviour, according to an OpenAI study on enhancing prompt engineering.
Consider the following two queries and their accompanying ChatGPT responses:
Prompt 1:

Prompt 2:
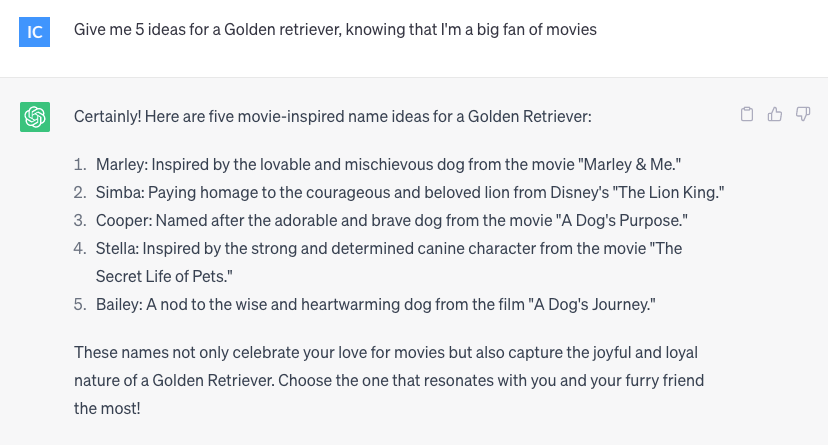
The second prompt yields a more particular and meaningful response.
This example highlights the significance of precision and clarity in writing prompts.
What are prompt categories?
Prompts are essential tools for facilitating seamless communication with AI language models.
To create high-quality prompts, you must first understand how they're classified. This lets you structure them effectively by focusing on a specific target response.
Major prompt categories include:
1. Information-seeking prompts
These prompts are crafted to gather information by posing "What" and "How" questions. They are ideal for extracting specific details or facts from the AI model. Examples include:
-
What are the health benefits of a plant-based diet?
-
How can I increase my productivity at work?
2. Instruction-based prompts
Instruction-based prompts direct the AI model to perform a specific task. These prompts resemble how we interact with voice assistants like Siri, Alexa, or Google Assistant. Examples include:
-
Schedule a dentist appointment for next Tuesday at 10 AM.
-
Find the fastest route to the airport.
3. Context-providing prompts
These prompts supply contextual information to the AI model, enabling it to comprehend the user's desired response better. By offering context, you can obtain more accurate and relevant answers from the AI. Examples include:
-
I'm new to gardening. What are some easy-to-grow plants for beginners?
-
I want to plan a romantic dinner for my partner. Can you suggest some recipes and ambience ideas?
4. Comparative prompts
Comparative prompts are utilized to evaluate or compare different options, assisting users in making informed decisions. They are particularly helpful when weighing the pros and cons of various alternatives. Examples include:
-
What are the benefits and drawbacks of renting versus buying a home?
-
Compare the performance of electric cars and traditional gasoline cars.
5. Opinion-seeking prompts
These prompts elicit the AI's opinion or viewpoint on a given topic. They can help generate creative ideas or engage in thought-provoking discussions. Examples include:
-
What could be the potential impact of artificial intelligence on the job market?
-
How might the world change if teleportation became a reality?
6. Reflective prompts
Reflective prompts help individuals gain deeper insights into themselves, their beliefs, and their actions. They often encourage self-growth and introspection based on a topic or personal experience. You may need to provide some background information to obtain a desirable response. Examples include:
-
How can I build my self-confidence and overcome self-doubt?
-
What strategies can I implement to maintain a healthy work-life balance?
What kind of factors influence prompt selection?
Prompt selection entails taking into account many elements to create effective prompts. These elements impact the quality, relevance, and accuracy of ChatGPT's responses. Essential factors to consider include:
-
Model knowledge: Learn about the advantages and disadvantages of ChatGPT. Even cutting-edge models like ChatGPT may need help with specific jobs or produce false data. This understanding aids in creating prompts that capitalise on the model's strengths while minimising its flaws.
-
User Intent: Understanding the user's intent to generate relevant responses is critical. The prompts should clearly reflect the user's expectations, allowing ChatGPT to give relevant and correct information.
-
Clarity and specificity: Make sure the prompt is clear and explicit to minimise ambiguity or uncertainty, which can lead to poor responses.
-
Domain specificity: When dealing with a highly specialised domain, consider employing domain-specific vocabulary or context to steer the model to the intended response. Adding context or examples can help the model produce more accurate and relevant results.
-
Limitations: Determine whether any limitations (such as response length or format) are required to produce the desired outcome. Constraints, such as character limitations or structured formats, can be explicitly specified to help the model generate responses that fit specific needs.
Considering these elements improves ChatGPT performance and guarantees that generated responses closely match the desired goals.
It is crucial to note that prompt engineering is an ongoing topic of study, with constant improvements and refinements being made to increase the interactivity and usefulness of language models such as Chat GPT.
To summarize:
Main prompting steps |
1. Identify the problem or goal: Clearly state the purpose you want ChatGPT to assist you with and the type of response you expect. Example: Generate catchy headlines for a marketing campaign targeting young professionals in the fashion industry. |
2. Add pertinent keywords and phrases: Include the most relevant industry-specific and topic-related terms in the prompt to help steer ChatGPT towards generating the desired output. Example: Craft persuasive marketing copy for our athletic shoes, focusing on comfort, durability, and performance. Target fitness enthusiasts and athletes. Incorporate keywords like 'performance,' 'comfort,' 'durability,' and 'athletic'. |
3. Compose the prompt: Construct a succinct prompt that effectively conveys the required information and task using the keywords and phrases identified in the previous steps. Example: "Create a compelling social media post highlighting the benefits of our new organic skincare line, emphasizing ingredients like 'natural', 'nourishing', 'eco-friendly', and 'glowing skin'." |
4. Test, assess, and iterate: Produce responses using ChatGPT, evaluate the outcomes, and modify the prompt as needed to obtain the desired output. Repeat this process until you create a successful prompt that consistently yields the expected response. |
What makes a good prompt |
Excellent outputs primarily depend on the training data, model parameters, and effective prompting. Since we can only influence one of these factors, here's what constitutes good prompting:
|
What are the techniques for effective prompt engineering?
Here we'll explore several techniques that can be employed to optimise prompts and maximise the effectiveness of interactions with ChatGPT. Let's delve into these techniques and understand their significance.
Clear and specific instructions
Clear and specific instructions form the foundation. By providing explicit guidance, users can improve the quality of ChatGPT's responses. Research conducted by OpenAI reveals that well-defined prompts significantly impact the performance of language models.
Prompt 1:
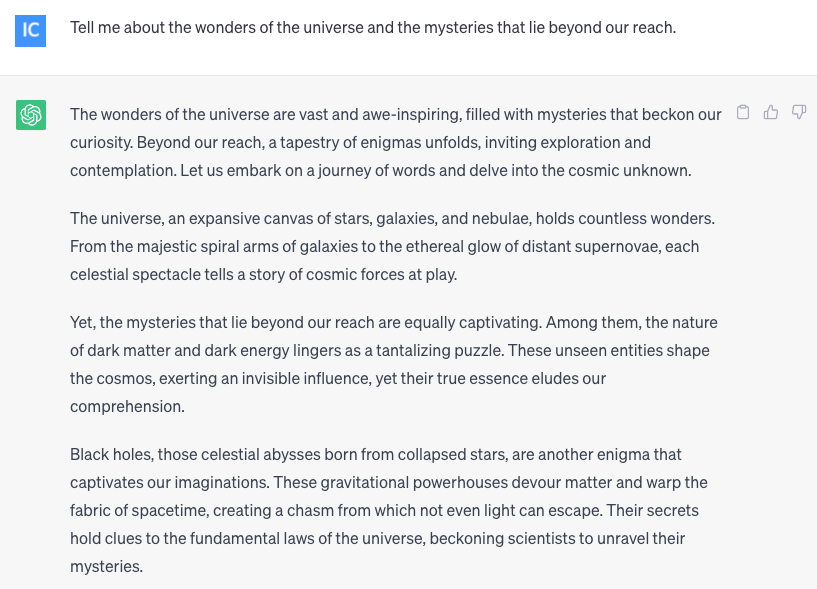
Prompt 2:

Using explicit constraints
Incorporating explicit constraints within prompts can guide ChatGPT's thinking process and ensure more accurate and reasoned responses. Constraints serve as additional instructions that shape the model's behaviour and improve the relevance of generated outputs.
For instance, when seeking step-by-step instructions, incorporating constraints such as "Please provide a detailed, sequential process" helps ChatGPT generate coherent, easy-to-follow instructions. OpenAI's research demonstrates that using explicit rules leads to more controlled and aligned outputs.
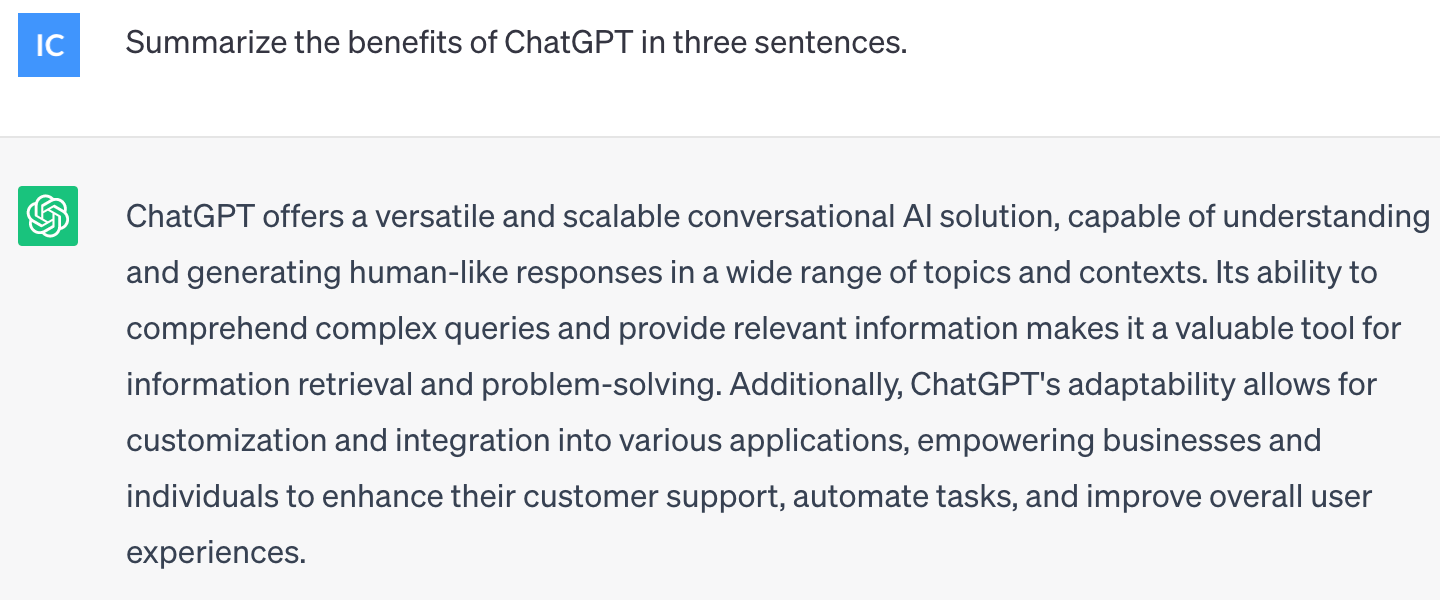
Experimenting with context and examples
Context plays a vital role in prompt engineering. By providing relevant context and examples within prompts, users can enhance ChatGPT's understanding and guide it towards generating more accurate and contextually appropriate responses.
For example, incorporating relevant context in the prompt helps ChatGPT provide more informed answers when requesting information about a specific topic.
Prompt 1:

Prompt 2:

This context-rich prompt guides ChatGPT to generate responses that align with the specific area of interest.
Leveraging System 1 and System 2 questions
System 1 and System 2 questions provide a balanced approach to prompt engineering. System 1 questions elicit quick, instinctive responses, while System 2 questions demand thoughtful, detailed answers. Combining both types of questions adds variety and depth to the interactions with ChatGPT.
So, leveraging System 1 and System 2 queries in quick engineering is an approach that can influence the type of responses given by ChatGPT.
Users can direct ChatGPT to generate responses that meet their needs by including System 1 and System 2 questions in the prompts. Consider the following example to demonstrate this concept:
Example: Travel Suggestions Chatbot
Prompt: "As a travel recommendations chatbot, you aim to provide users with personalised and informed travel suggestions. Use both System 1 and System 2 questions to generate relevant responses. System 1 questions should focus on quick recommendations, while System 2 questions should explore the reasoning behind the suggestions."
System 1 question: "What are some popular tourist attractions in Paris?"
System 2 question: "Can you explain the historical significance of the Eiffel Tower and its architectural features?"
The System 1 query in this example prompts ChatGPT to deliver fast recommendations for major tourist spots in Paris. Users looking for short travel itinerary advice would benefit from brief and easily digested information. Attractions such as the Louvre Museum, Notre Dame Cathedral, and the Champs-Élysées could be included in the response.
ChatGPT is encouraged by the System 2 inquiry to explore a particular monument's historical relevance and architectural aspects, such as the Eiffel Tower. This response would be helpful to users looking for a better understanding and insights about the attraction. The answer could include details on the tower's construction for the World's Fair in 1889, Gustave Eiffel's design, and its famous iron lattice framework.
Users can obtain rapid recommendations and more extensive explanations by including System 1 and System 2 inquiries. This enables the travel recommendations chatbot to adapt to various user tastes, delivering practical suggestions while also fulfilling the curiosity of individuals interested in the historical and architectural features of the sites.
Controlling output verbosity
Controlling the verbosity of ChatGPT responses is a critical component of quick engineering. It allows customers to control the level of detail and length of the generated outputs. Consider the following example to see how output verbosity can be managed:
Prompt 1:

Prompt 2:
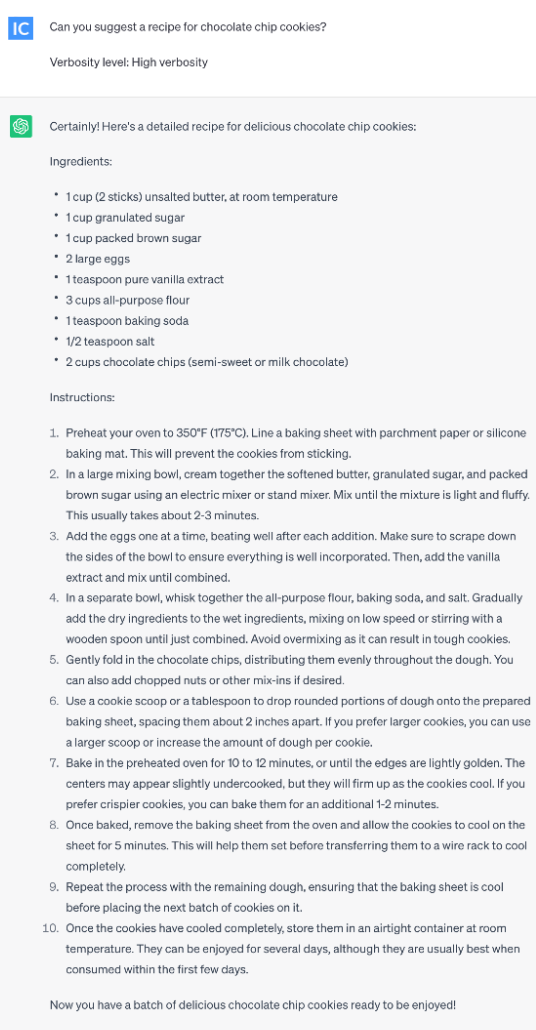
The chatbot demonstrates great verbosity in this response by presenting a detailed step-by-step recipe. It contains additional explanations and advice to help users navigate the baking process. This level of depth is appropriate for people who desire detailed instructions, particularly those new to baking or who appreciate a more comprehensive approach.
This way, the chatbot can adapt to diverse user preferences and optimise the conversation experience by regulating the verbosity of the responses, allowing the chatbot to provide relevant answers to their needs and improve their connection.
What are prompt engineering best practices?
Prompt engineering is a dynamic process that involves considerable thought and experimentation to attain the best results. In this section, we will look at best practices to assist users in getting the most out of their interactions with ChatGPT. Let's look into these practices and see what they mean.
Iterative testing and improvement
Effective prompts rely heavily on iterative testing and refinement. Users can refine the instructions and increase the quality of ChatGPT's responses over time by regularly reviewing the outputs and adjusting the prompts accordingly.
Iteration 1:

Iteration 2:
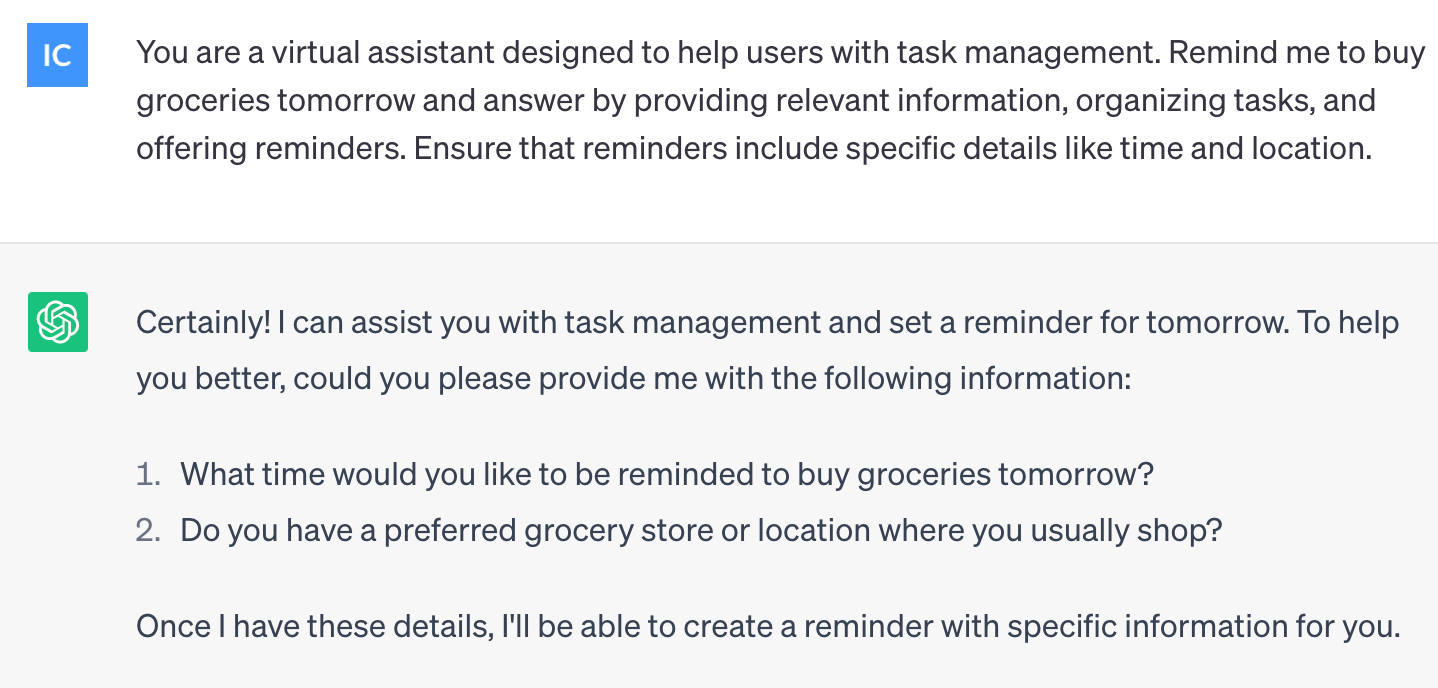
Iteration 3:
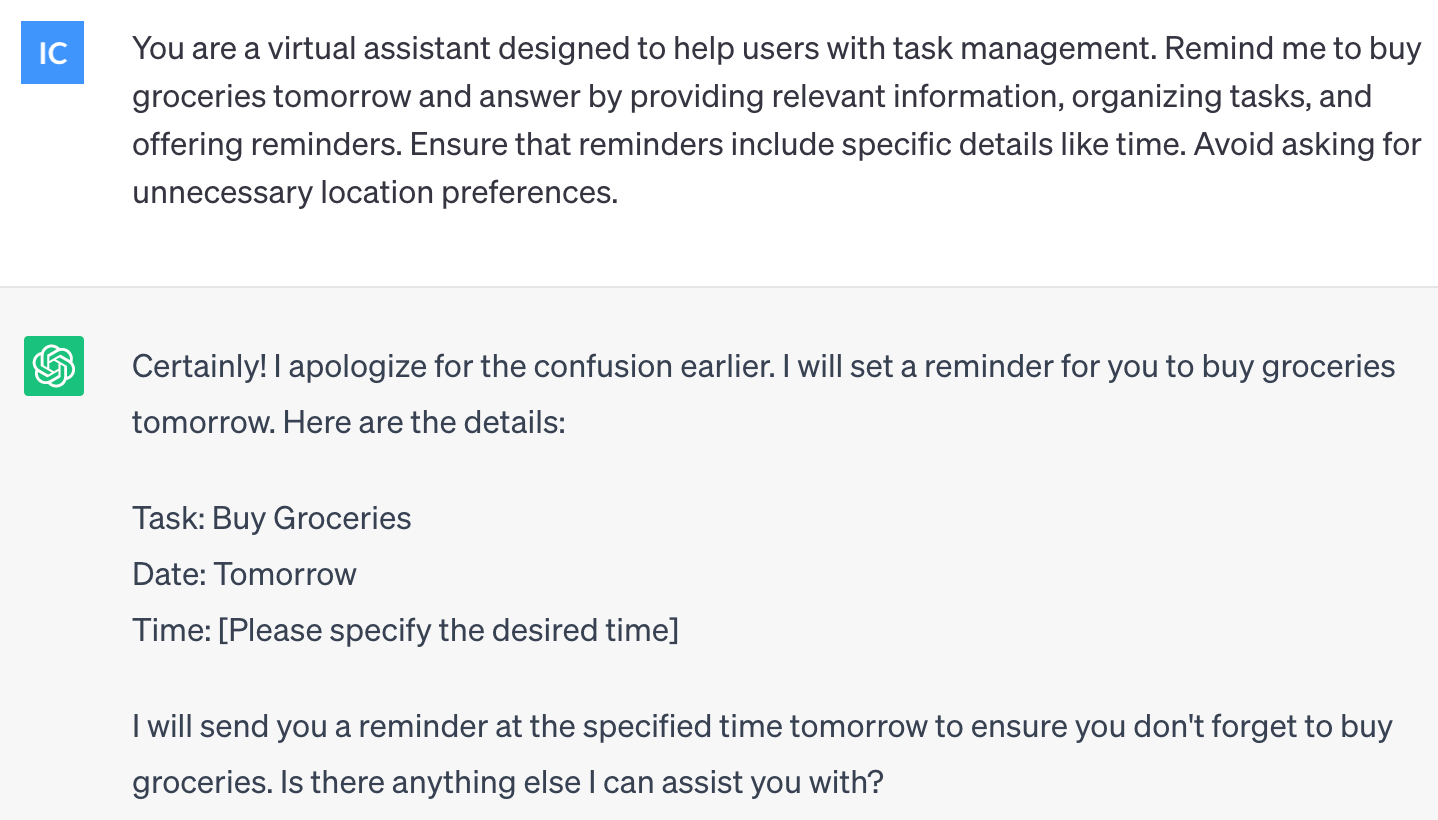
Achieving a balance between user intent and model creativity
Balancing user intent with model creativity is critical. Consider the following writing inspiration chatbot, which strives to deliver appropriate prompts while promoting creativity:
User query: "I need ideas for a fantasy story."
Prompt: "Imagine a world where magic is intricately woven into everyday life. Describe an individual with distinct magical power and their quest to safeguard a long-lost artefact. Use this prompt to inspire your own imaginative story."
Response: "A young sorcerer named Elysia possesses the rare ability to manipulate the elements in a magical world. She embarks on a perilous journey to protect a powerful artefact from dark forces. Along the way, she encounters diverse characters with their magical talents, unravelling mysteries and saving their world from impending doom."
The chatbot gives appropriate prompts that encourage imagination while remaining consistent with the user's writing goals by balancing matching user intent and boosting model creativity.
Making use of external resources and APIs
Harnessing external resources and APIs is a powerful technique that enables ChatGPT to leverage additional information and enhance its responses. Let's explore an example to understand how external resources and APIs can be used:
Example: Weather Information Chatbot
Prompt: "As a weather information chatbot, harness external resources and APIs to provide accurate and up-to-date weather data to users."
User query: "What's the weather like in New York City today?"
The chatbot can integrate with an external weather API, such as Weather.com, to provide accurate weather information. The chatbot can retrieve real-time weather data for the specified location by making an API call.
API Integration:
import requests
def get_weather(city):
api_key = "YOUR_API_KEY"
url = f"https://api.weatherapi.com/v1/current.json?key={api_key}&q={city}"
response = requests.get(url)
data = response.json()
return data["current"]["condition"]["text"], data["current"]["temp_c"]
The get_weather()
function above demonstrates an example integration with the WeatherAPI, which returns the weather condition and temperature in Celsius for a given city.
Response Generation:
city = "New York City"
weather_condition, temperature = get_weather(city)
response = f"The weather in {city} today is {weather_condition}. The temperature is {temperature}°C."
Generated Response:
"The weather in New York City today is partly cloudy. The temperature is 22°C."
By harnessing external resources and APIs, the chatbot retrieves accurate weather information and incorporates it into the response. This provides users with real-time weather updates tailored to their specified location.
Integration with external resources and APIs allows ChatGPT to tap into a wealth of information beyond its training data, enabling it to provide more valuable and reliable responses to user queries.
OpenAI API Example
This API allows developers to integrate ChatGPT into their applications, products, or services. Here's an example that showcases how the OpenAI API can be used:
import openai
# Set up your API credentials
openai.api_key = 'YOUR_API_KEY'
# Define a function for interacting with ChatGPT
def ask_chatbot(question, chat_history=None):
if chat_history is None:
chat_history = []
# Format the input for the API call
input_text = '\n'.join(chat_history + [f'User: {question}'])
# Call the OpenAI API to generate a response
response = openai.Completion.create(
engine='text-davinci-003',
prompt=input_text,
max_tokens=50,
temperature=0.7,
n=1,
stop=None,
temperature=0.7
)
# Extract the generated response from the API response
answer = response.choices[0].text.strip()
# Append the user's question and the chatbot's answer to the chat history
chat_history.append(f'User: {question}')
chat_history.append(f'Chatbot: {answer}')
return answer
# Example usage
chat_history = []
user_question = "What is the capital of France?"
response = ask_chatbot(user_question, chat_history)
print(response)
In this example, we define the ask_chatbot()
function, which inputs a user's question and an optional chat history. The function formats the chat history and user questions and then makes an API call using the openai.Completion.create()
method.
The API response contains the generated response from ChatGPT. We extract the answer from the response and append the user's question and the chatbot's answer to the chat history. Finally, the generated answer is returned.
By using this API, developers can integrate ChatGPT's capabilities into their applications, allowing users to interact with the chatbot and receive responses based on their queries.
Avoiding biases and ensuring ethical usage
ChatGPT must be used ethically and without bias. An example of these practises' importance:
Example: AI-Powered Job Candidate Screening
Imagine an AI system that analyses interview responses using ChatGPT to screen job candidates. Screening must be ethical and bias-free.
These steps can reduce bias and assure fairness:
-
Diverse training data: Use various race, gender, and ethnicity data to fine-tune ChatGPT. Addressing biased training data from the start is essential because it can cause biases.
-
Bias evaluation: Regularly evaluate model responses to identify and reduce biases. Use demographic parity and equal opportunity to see if the model's suggestions vary by protected factors like gender or race. Adjustments should reduce biases.
-
Transparent guidelines: Communicate system guidelines to human reviewers and developers. These standards should stress justice, ethics, and bias avoidance. Give explicit instructions to avoid favouring or discriminating against specific groups during screening.
-
Human-in-the-loop review: Have humans analyse the system's replies. This stage helps catch any model biases and guarantees that humans can consider the context and make fair decisions.
-
Ongoing monitoring and feedback: Continuously monitor the system's performance and collect feedback from users and reviewers. Check system outputs for biases and unexpected effects. Feedback loops help resolve difficulties quickly.
-
Diverse reviewer pool: Ensure that the team responsible for reviewing and refining the system's outputs consists of individuals from diverse backgrounds. Diverse groups can spot and resolve prejudices that homogenous groups miss.
These practices help the AI-powered job candidate screening system avoid prejudices and evaluate candidates based on their skills and qualifications.
What are advanced prompt engineering strategies?
Prompt engineering goes beyond the basics to include innovative tactics for further optimising ChatGPT's performance and adaptability. This section looks at advanced strategies, such as temperature and token control, prompt chaining for multi-turn talks, modifying prompts for domain-specific applications, and dealing with confusing or contradicting user inputs.
Temperature and token management
Temperature and token control are effective methods for fine-tuning ChatGPT behaviour. Users can change the randomness of the generated output using temperature control. Lower temperatures, such as 0.2, create more focused and deterministic answers, whereas higher temperatures, such as 1.0, produce more variable and exploratory results.
OpenAI research reveals the effect of temperature control on ChatGPT response diversity. Users can achieve the ideal mix between offering comprehensible answers and incorporating fresh features into the generated responses by experimenting with different temperature settings.
Prompt 1:
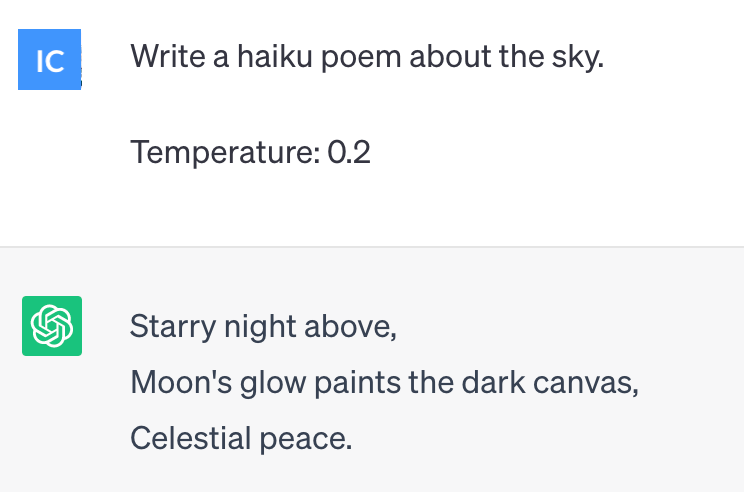
Prompt 2:

Token control requires specifying the maximum number of tokens to limit the length of the answer. This allows users to control the verbosity of ChatGPT's output and receive brief and to-the-point responses. Users can verify that ChatGPT provides responses that correspond to their desired response length by establishing appropriate token limitations.
Prompt chaining and multi-turn conversations
Prompt chaining and multi-turn conversations enable more interactive and dynamic interactions with ChatGPT. Instead of relying on single prompts, users can chain prompts together to create a continuous flow of conversation. Each prompt can reference previous inputs or ChatGPT's previous responses, allowing for a contextually rich conversation.
By incorporating prompt chaining, users can create a more conversational experience and engage in back-and-forth interactions with ChatGPT. This technique benefits tasks requiring multi-step instructions or engaging in detailed discussions.
Example:
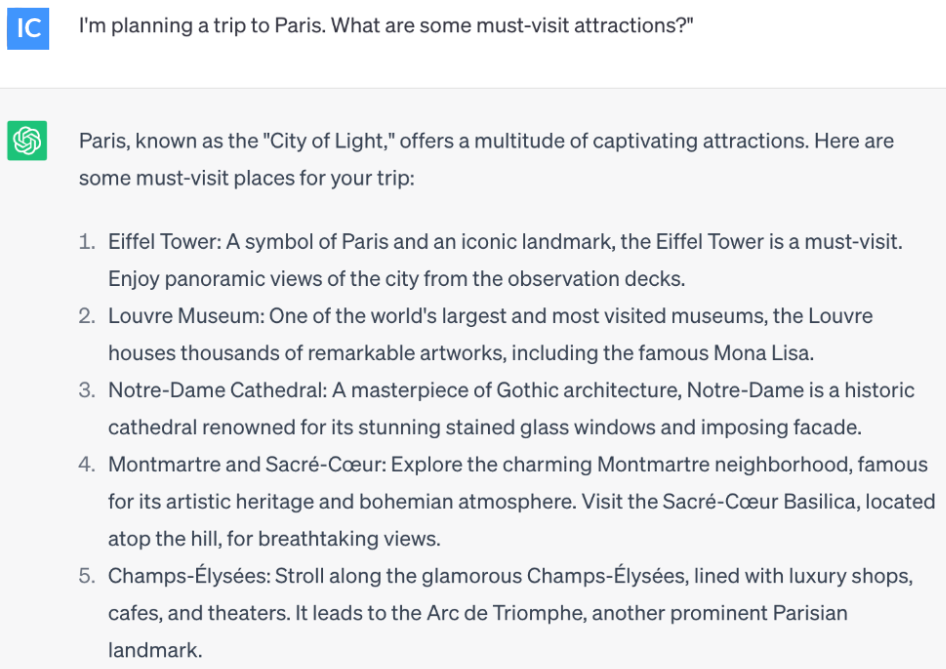
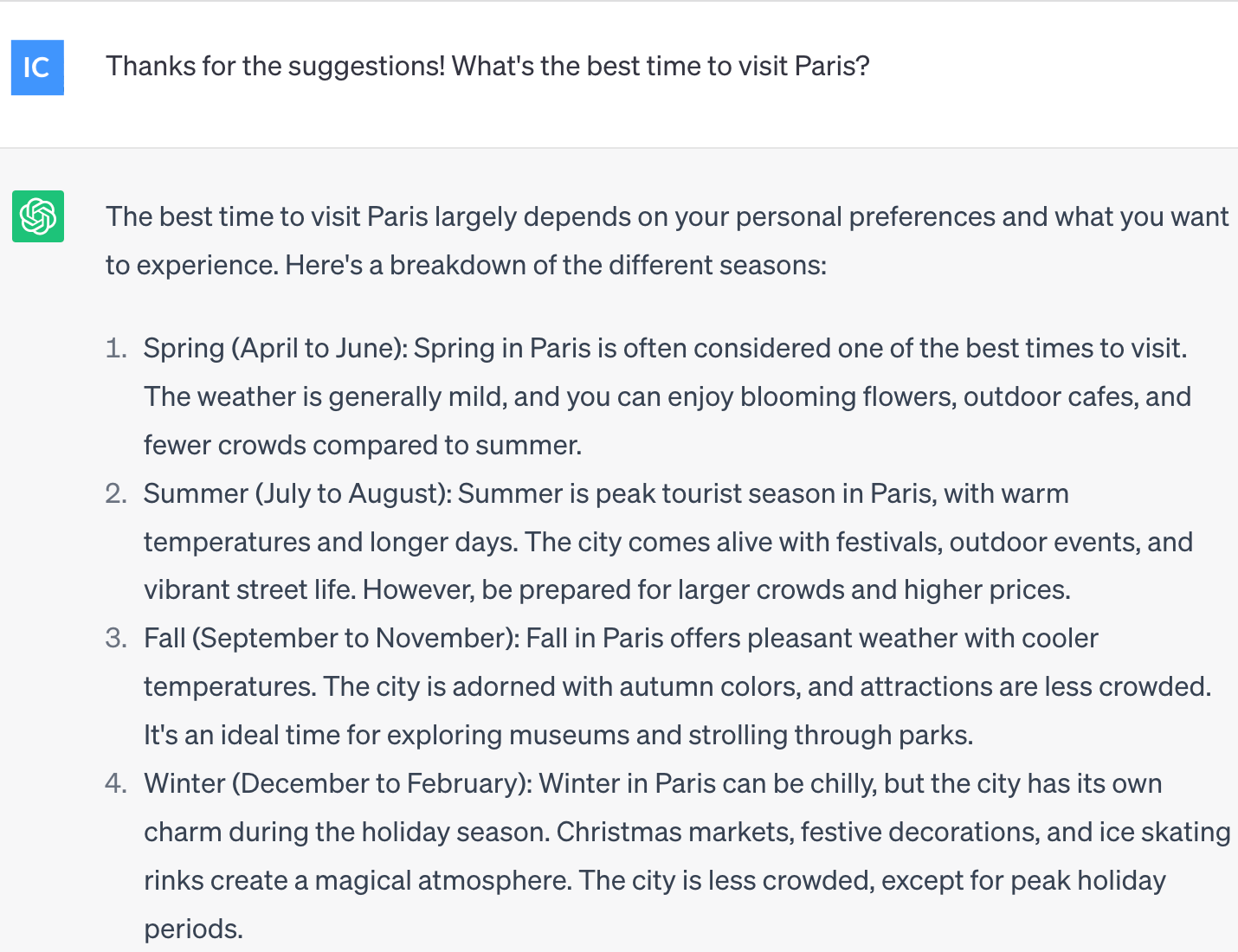
Adapting prompts for domain-specific applications
Adapting prompts for domain-specific applications is an essential aspect of prompt engineering. It involves tailoring the prompts to specific industries or fields to ensure relevant and accurate responses. Let's explore an example to illustrate how prompts can be adapted for a domain-specific application:
Example: Medical Diagnosis Chatbot
Prompt: "Develop a prompt for a medical diagnosis chatbot that helps users assess their symptoms and provides initial recommendations."
Adapting the prompt for a medical diagnosis chatbot requires incorporating relevant medical terminology, symptoms, and diagnostic considerations.
Prompt: "Describe your symptoms and medical history, and the chatbot will provide an initial assessment based on the information provided. Please note that this assessment is not a substitute for professional medical advice. If you are experiencing a medical emergency, please contact emergency services immediately."
User query: "I have a headache, fever, and sore throat."
The adapted prompt considers the user's symptoms and informs them about the limitations of the assessment. The chatbot-generated response can provide initial recommendations based on the information provided:
Response: "Based on your symptoms of headache, fever, and sore throat, it could indicate a common viral infection such as the flu or a possible strep throat. Consulting a healthcare professional for a definitive diagnosis and appropriate treatment is important. In the meantime, you can consider getting rest, staying hydrated, and taking over-the-counter pain relievers to alleviate your symptoms. If your symptoms worsen or persist, it is advisable to seek medical attention for further evaluation."
By adapting the prompt to a medical diagnosis chatbot, the response aligns with the domain-specific application and provides initial recommendations while emphasising the importance of professional medical advice.
Handling ambiguous or contradictory user inputs
Prompt engineering requires handling unclear or contradicting user inputs. ChatGPT must carefully handle such inputs and respond meaningfully. Let's explore an example to illustrate how this can be achieved:
Example: Restaurant Recommendation Chatbot
Prompt: "As a restaurant recommendation chatbot, handle ambiguous or contradictory user inputs to ensure accurate and helpful responses."
User query: "I'm looking for a restaurant in the city with vegetarian and steak options."
In this case, the user wants steak and vegetarian options. The chatbot can clarify the following:
Response: "Yes! I'd be happy to help you find a restaurant. What's your preference? Are you leaning more towards a vegetarian-focused establishment or one that offers a variety of options, including steaks?"
The chatbot requests clarification to understand the user's request better and deliver a more accurate recommendation.
User response: "I like a restaurant with vegetarian and steak dishes."
After the user specifies their preference, the chatbot can respond:
Response: "Great! I recommend "The Grill House" for its vegetarian and meat dishes. They serve delicious vegetarian and beef dishes on a varied menu. Enjoy your dining experience!"
By actively engaging with the customer and seeking clarification, the chatbot manages the initial query's ambiguity, understands the user's desire, and recommends a restaurant that matches their request.
Handling conflicting user inputs is similar. The chatbot can clarify the user's goals and provide a solution if they want a cheap but luxurious meal.
Case studies
Here are some case examples to examine.
Customer support chatbots
Client service chatbots improve customer service and reaction time. Prompt engineering can increase chatbot accuracy and efficiency, enhancing customer experiences.
It helps chatbots learn and respond to client inputs, making interactions more personalised and effective.
Example: HubSpot Chatbot Builder, which can book meetings, link to self-service support articles, and integrate with a ticketing system
Content creation and editing
Content creation and editing require prompt engineering. ChatGPT helps users write great blog posts, emails, and creative pieces.
Users can assist ChatGPT in developing text that matches their style, tone, and goal by providing specific and detailed prompts. Prompts can offer background, examples, or explicit limits to ensure generated content fulfils criteria.
OpenAI studied prompt engineering to improve content coherence and relevancy, so users generated more engaging and on-topic text by experimenting with suggestions, and saving editing time.
Domain-specific knowledge retrieval
Prompt engineering can efficiently retrieve domain-specific knowledge. ChatGPT may be trained on enormous amounts of domain-specific data to offer accurate and relevant subject information.
Users can prompt ChatGPT to retrieve domain-specific knowledge by customising prompts and adding keywords or context. The correct information is essential in industries like healthcare, law, finance, and technology.
Its strategies promote domain-specific knowledge retrieval, giving consumers accurate and up-to-date information.
Interactive storytelling and gaming
Prompt engineering makes interactive storytelling and games exciting. ChatGPT responds to user inputs and drives the story.
Users can construct immersive stories and games using prompts introducing tale components, user choices, or game mechanisms. Prompt chaining and multi-turn discussions enable rich narratives and gaming interactions.
Example: OpenAI's AI Dungeon shows how prompt engineering may change interactive storytelling and gaming. AI Dungeon lets users collaborate on dynamic narratives via prompts.
ChatGPT prompt engineering for developers
Deep Learning AI recently launched an exceptional course called "ChatGPT Prompt Engineering for Developers," led by Isa Fulford and Andrew Ng.
During the course, they emphasize that the potential of Large Language Models (LLMs) as a developer tool, utilizing API calls to LLMs for swift software application development, is still underappreciated. They aim to share the possibilities and best practices for leveraging LLMs effectively. The course covers prompting best practices for software development, everyday use cases such as summarization, inference, transformation, and expansion, and building a chatbot using an LLM.
OpenAI's chatGPT model, specifically GPT 3.5 Turbo, and Python (particularly in a Jupyter Notebook) are utilized throughout the course.
So here are some learnings:
1. Two Principles:
Principle 1: Write Clear and Specific Instructions
It is crucial to express clear and specific instructions to guide the model effectively and reduce the likelihood of irrelevant or incorrect responses. Avoid confusing a clear prompt with a short one, as longer prompts often provide more clarity and context, leading to detailed and relevant outputs.
- Tactic 1: Use delimiters to indicate distinct parts of the input, such as triple quotes ('''), triple backticks ('*'), triple dashes ('---'), angle brackets (‹ ›), or XML tags (‹tag› ‹/tag›). Delimiters also help prevent prompt injections, where conflicting user instructions may misdirect the model.
text = f"""
You should express what you want a model to do by \
providing instructions that are as clear and \
specific as you can possibly make them. \
This will guide the model towards the desired output, \
and reduce the chances of receiving irrelevant \
or incorrect responses. Don't confuse writing a \
clear prompt with writing a short prompt. \
In many cases, longer prompts provide more clarity \
and context for the model, which can lead to \
more detailed and relevant outputs.
"""
prompt = f"""
Summarize the text delimited by triple backticks \
into a single sentence.
```{text}```
"""
- Tactic 2: Request structured output like HTML or JSON for easier parsing of model responses.
prompt = f"""
Generate a list of three made-up book titles along \ with their authors and genres.
Provide them in JSON format with the following keys: book_id, title, author, genre.
"""
response = get completion (prompt)
print (response)
- Tactic 3: Verify whether the task assumptions are satisfied. Prompt the model to check these assumptions first and indicate any unsatisfied conditions without attempting a full task completion. Consider potential edge cases to ensure the model handles them appropriately and avoids unexpected errors or results.
- Tactic 4: Utilize few-shot prompting by providing examples of successfully executed tasks before asking the model to perform the desired task.
prompt = f"""
Your task is to answer in a consistent style.
<child>: Teach me about patience.
<grandparent>: The river that carves the deepest \ valley flows from a modest spring; the \ grandest symphony originates from a single note; \ the most intricate tapestry begins with a solitary thread.
<child>: Teach me about resilience.
"""
response = get completion (prompt)
print (response)
Principle 2: Give the Model Time to Think
Allow the model sufficient time to think and reason through the problem to prevent reasoning errors and premature conclusions. Complex tasks may require step-by-step instructions or a chain of relevant reasoning before the model provides a final answer.
- Tactic 1: Specify the steps to complete a task, especially when direct answers are challenging. Like human problem-solving, request the model to engage in a series of appropriate reasoning steps before delivering the final solution.
prompt 2 = f"""
Your task is to perform the following actions:
1 - Summarize the following text delimited by <> with 1 sentence.
2 - Translate the summary into French.
3 - List each name in the French summary.
4 - Output a json object that contains the following keys: french_ summary, num names.
Use the following format:
Text: <text to summarize>
Summary: <summary>
Translation: <summary translation>
Names: <list of names in Italian summary>
Output JSON: <ison with summary and num names>
Text: <{text!>
response = get_completion (prompt_2)
print "InCompletion for prompt 2:")
print (response)
- Tactic 2: Instruct the model to find its solution before reaching a conclusion. Explicitly instructing the model to reason and deliberate before providing an answer often yields better results. This approach allows the model time to process and derive accurate responses.
By following these principles and tactics, developers can optimize their use of LLMs and achieve desired outcomes in software development.
2. Iterative Prompt Development:
The process of iterative prompt development closely resembles coding practices. It involves trying different approaches, refining and retrying as needed. Here are the steps involved:
- Attempt a solution.
- Analyze the results to identify any discrepancies from the desired outcome.
- Clarify instructions and allow more time for deliberation.
- Refine prompts using a batch of examples.
- Repeat the process.
In the course example, the instructors presented a case study on generating marketing copy from a product fact sheet. They iteratively addressed and resolved three critical issues by refining prompts at each step:
Issue 1: Lengthy text -> Solution: Limit the text to a maximum of 50 words.
Issue 2: Focus on irrelevant details -> Solution: Incorporate intended audiences, such as "The description is intended for furniture retailers..."
Issue 3: Lack of dimensions table in the description -> Solution: Format everything as HTML.
3. Capabilities:
Summarizing:
Large Language Models have been widely employed for text summarization. You can request summaries focusing on price and value by providing specific prompts.
prompt = f"""
Your task is to generate a short summary of a product \
review from an ecommerce site to give feedback to the \
pricing deparmtment, responsible for determining the \
price of the product.
Summarize the review below, delimited by triple
backticks, in at most 30 words, and focusing on any aspects \
that are relevant to the price and perceived value.
Review: ```{prod_review}```
"""
And you can also write a for loop to summarize multiple texts:
reviews = [review_1, review_2, review_3, review_4]
for i in range(len(reviews)):
prompt = f"""
Your task is to generate a short summary of a product \
review from an ecommerce site.
Summarize the review below, delimited by triple \
backticks in at most 20 words.
Review: ```{reviews[i]}```
"""
response = get_completion(prompt)
print(i, response, "\n")
Inferring:
LLMs can infer various aspects without specific training. They can determine sentiment, emotions, extract product and company names, figure topics, and more.
Transforming:
LLMs excel in text transformation tasks, including language translation, spelling and grammar checking, tone adjustment, and format conversion.
prompt = f"""
Translate the following text to French and Spanish and English pirate: \
```I want to order a basketball```
"""
response = get completion (prompt)
print response
Expanding:
Large Language Models can generate personalized customer service emails tailored to each customer's review.
prompt = f"""
You are a customer service AI assistant.
Your task is to send an email reply to a valued customer.
Given the customer email delimited by ```,\
Generate a reply to thank the customer for their review.
If the sentiment is positive or neutral, thank them for \
their review.
If the sentiment is negative, apologize and suggest that \
they can reach out to customer service.
Make sure to use specific details from the review.
Write in a concise and professional tone.
Sign the email as `AI customer agent`.
Customer review: ```{review}```
Review sentiment: (sentiment}
"""
response = get completion (prompt, temperature=0.7)
print(response)
Developing a Chatbot:
One of the fascinating aspects of using a LLM is the ability to create a customized chatbot effortlessly. ChatGPT's web interface offers a conversational platform enabled by a robust language model. However, the real excitement lies in harnessing the capabilities of a LLM to construct your chatbots, such as an AI customer service agent or an AI order taker for a restaurant.
In this case, we'll refer to the chatbot as "OrderBot." The aim is to automate collecting user prompts and assistant responses to construct this efficient "OrderBot." Primarily designed for taking orders at a pizza restaurant, the initial step involves defining a useful function. This function facilitates the collection of user messages, eliminating the need for manual input. The prompts gathered from a user interface created below are then appended to a list called "context." Subsequently, the model is invoked with this context for each interaction.
The model's response is incorporated into the context, ensuring that both the model's and the user's messages are retained, contributing to the growing context. This accumulation of information empowers the model to determine the appropriate actions to take.
Finally, the user interface is set up and executed to display the OrderBot. The context, which includes the system message containing the menu, remains consistent across each interaction with the language model. It steadily evolves as more interactions occur, maintaining a comprehensive conversation record.
Final thoughts
Prompt engineering is a game-changer for ChatGPT. By mastering this technique, you can shape and guide the responses of the language model to meet your specific needs.
The future looks promising, with ongoing research and collaboration driving innovation. As language models evolve, prompt engineering will play a pivotal role in harnessing their full potential.
ChatGPT's prompt engineering opens unlimited options. We can transform our interactions with language models by implementing effective techniques and exploring advanced strategies. It transforms customer care chatbots, content development, and games, enabling human-AI collaboration.
If you want to learn more about our data science services, including AI and Natural Language Processing (NLP), we invite you to explore the Imaginary Cloud AI website. We are experts at providing AI-driven solutions to help businesses harness the power of artificial intelligence.
FAQs
What is prompt engineering in ChatGPT?
Prompt engineering is the process of designing effective prompts and instructions to communicate user intent to a language model like ChatGPT. It helps in obtaining accurate, relevant, and useful responses from the model.
Why is prompt engineering important for ChatGPT?
Prompt engineering is crucial for maximizing the effectiveness of ChatGPT. By crafting well-designed prompts, users can guide the model to generate more accurate and relevant outputs, making it a valuable tool for various applications.
What are some techniques for effective prompt engineering?
Techniques include:
- Providing clear and specific instructions
- Using explicit constraints
- Experimenting with context and examples
- Leveraging System 1 and System 2 questions
- Controlling output verbosity
How can I improve my prompts for better ChatGPT performance?
To improve your prompts, you can:
- Test and refine them iteratively
- Balance user intent and model creativity
- Use external resources and APIs to enhance ChatGPT's capabilities
- Ensure ethical usage and avoid biases in both prompts and outputs
Here's also a ChatGPT cheat sheet to help you write good performing prompts to begin.
What are some advanced strategies for prompt engineering?
Advanced strategies include:
- Controlling temperature and token settings for randomness and response length
- Creating multi-turn conversations through prompt chaining
- Adapting prompts for domain-specific applications
- Handling ambiguous or contradictory user inputs
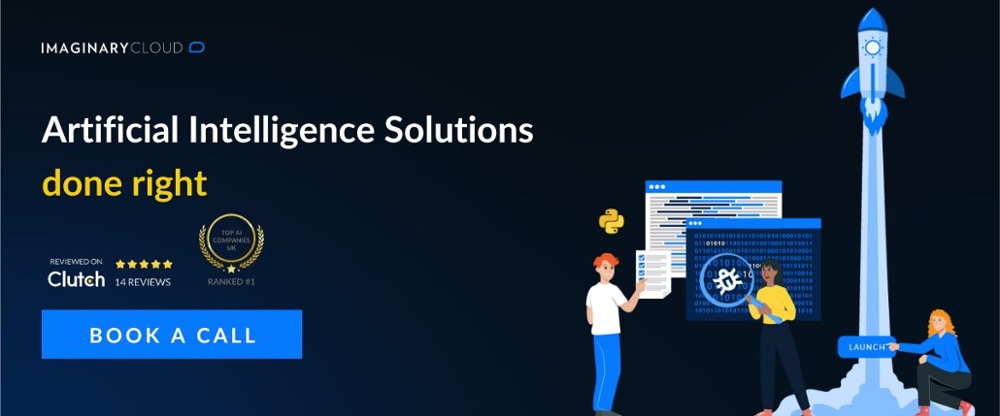